Reduce Time to Market & Speed up Production Launches by Increasing Gage Study Efficiency
When testing chips in production, measurement system error is your enemy. It can make a good part look bad, resulting in lower yields as you throw away parts that are actually good. Or, even worse, it can make a bad part look good, resulting in a field failure which is costly in many ways.
Since a gage study is a gating item to production testing, spending less time running and debugging after the results of a gage study can get you to market faster, giving you a leg up on competition, and increasing your bottom line!
The most common method of understanding and improving your measurement system error is by running a gage study. This analyzes the repeatability (how accurate can the same system measure the same part) and reproducibility (how accurate can different testers, loadboards, sites, etc measure the same part) of your measurement system. The main metric used for the result of the gage study is an R&R % which is a function of your repeatability and reproducibility. Generally speaking, if it’s over 30% it needs to be investigated further, and resolved if possible. However, many industries use gage studies, and many of the measurements taken don’t have any spec limits. In those cases the R&R % is the golden standard. However in the product and test engineering world, we have test limits for the parameters we are measuring and we can use this as an advantage in gage studies to reduce chasing our tails, debugging time, and getting to market faster!
What if I told you this test had an R&R% of 46% mainly due to the tester to tester reproducibility? Since it’s over the 30% threshold, you’d need to spend a lot of time debugging, and trying to fix the issue with experimenting with new matching components on the board, or wait time / methodology changes in how the test was run.
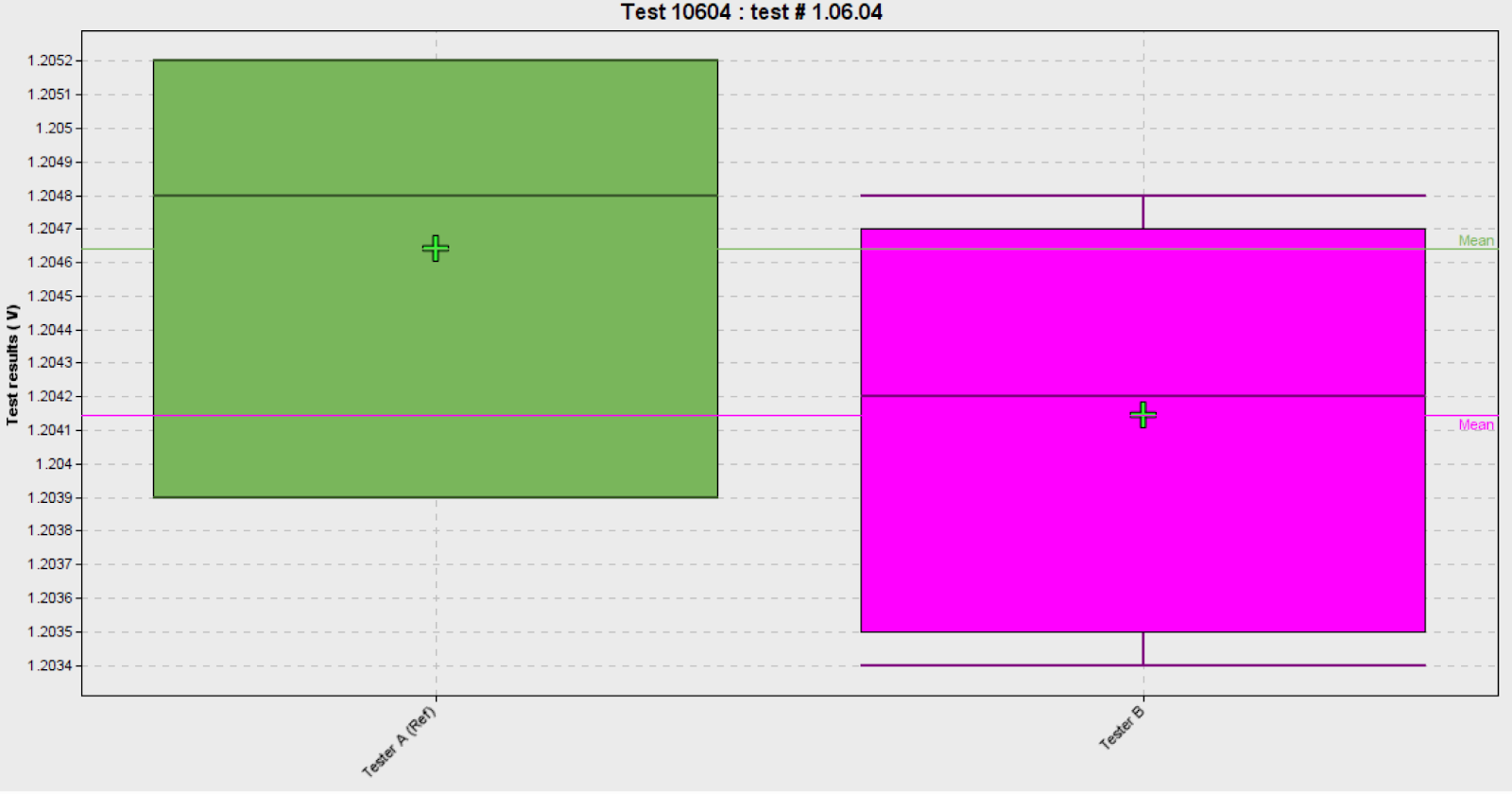
But we have the luxury of having test limits! What if I zoomed out, and showed you where our typical measurements are in relation to the actual test limits?

You can barely see a difference in the results now, and in relation to the test limits (with a cpk of roughly 15) do you think the tester to tester reproducibility would be an issue in production? Absolutely not! Since we have this luxury of having test limits, we can use a more meaningful metric called the Precision to Tolerance ratio (P/T%). This is very close to the Gage R&R formula but instead of dividing the R&R (repeatability and reproducibility) by the limitless total variation, it divides it by your limit space (upper limit - lower limit). So if the R&R% was an alarming 46% (over the 30% threshold needed to invest time into debugging the “issue”), what do you think the P/T% is? Go ahead, make a guess! Well, it’s a whopping 1.81%!
What does this mean to you as a product or test engineer? It means you can spend less time chasing your tail debugging your gage study results for “issues” that aren’t really there since you have the luxury of test limits and performance results. In fact in this part’s data, a normal Gage R&R% flagged 53 tests as being over the 30% threshold whereas the P/T ratio only flagged 1! That could save you weeks, or even months of wasted debugging that isn’t necessary!
Gage studies can be performed using many different tools. When doing so, use the P/T ratio with your data that has test limits and then go enjoy all that extra time not spent debugging on the beach! Galaxy’s Examinator also has the ability to run gage studies with either R&R% or the P/T% so you can see the difference for yourself, and you can start a free trial today! Click here to learn more! https://www.galaxysemi.com/products/examinatorpro